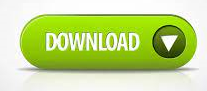

Besides, macro-economic factors like a growing population and improvement in the overall economy growth rate also play their part in the growth of this market. Increasing disposable income also plays a major part in the growth of steep slope roofing materials market. The demand of steep slope roofing materials is tied to the growth in the construction industry. In addition, another main advantage of building a steep slope roof is that they accelerate the water run-off rate, due to which water doesn’t tend to accumulate on the roof and the build-up of debris and mold is avoided. Also, they can be transformed into wide variety of shapes, giving a vast scope to creativity and new design techniques. The steep slope roofs possess the characteristics of greater aesthetic appeal, long service life, resistance to wind and low maintenance requirements. In other words, steep slope roofs are attractive and even affordable. Steep slope roofs are preferred by both the customers and architects since they add aesthetic appeal to the building and even offer a long-term performance. This translates into for every 12 horizontal inches, the rise of the roof is 3 inches or more. The code and pre-trained trustworthiness predictors for reproducibility are available at \url.A steep slope roofing is defined as roofing that are suitable for roofs possessing slopes of 3:12 or greater. We conduct comprehensive experiments and analyses on ImageNet, which show that the proposed loss effectively improves the generalizability of trustworthiness predictors. The proposed loss is evaluated with two representative deep learning models, i.e., Vision Transformer and ResNet, as trustworthiness predictors. incorrect predictions by two slide-like curves that oppose each other. To improve the generalizability of trustworthiness predictors, we propose a novel steep slope loss to separate the features w.r.t. Secondly, due to the data complexity, it is challenging to differentiate the incorrect predictions from the correct ones on real-world large-scale datasets. Firstly, correct predictions are generally dominant over incorrect predictions. In such a setting, we observe that the trustworthiness predictors trained with prior-art loss functions, i.e., the cross entropy loss, focal loss, and true class probability confidence loss, are prone to view both correct predictions and incorrect predictions to be trustworthy. In this work, we study the problem of predicting trustworthiness on real-world large-scale datasets, where the task is more challenging due to high-dimensional features, diverse visual concepts, and a large number of samples. Prior efforts have been proven to be reliable on small-scale datasets. Abstract: Understanding the trustworthiness of a prediction yielded by a classifier is critical for the safe and effective use of AI models.
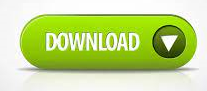